Ways To Analyse Logs Using The File
In today’s technology-driven world, logs play a crucial role in understanding and troubleshooting system issues. Logs are invaluable sources of information that record events, errors, and activities within an application or system. Analyzing logs can provide valuable insights into system performance, identify potential security breaches, and aid in debugging. In this blog, we will explore six effective ways to analyze logs using the file, empowering you to extract meaningful information and improve your system’s overall performance.
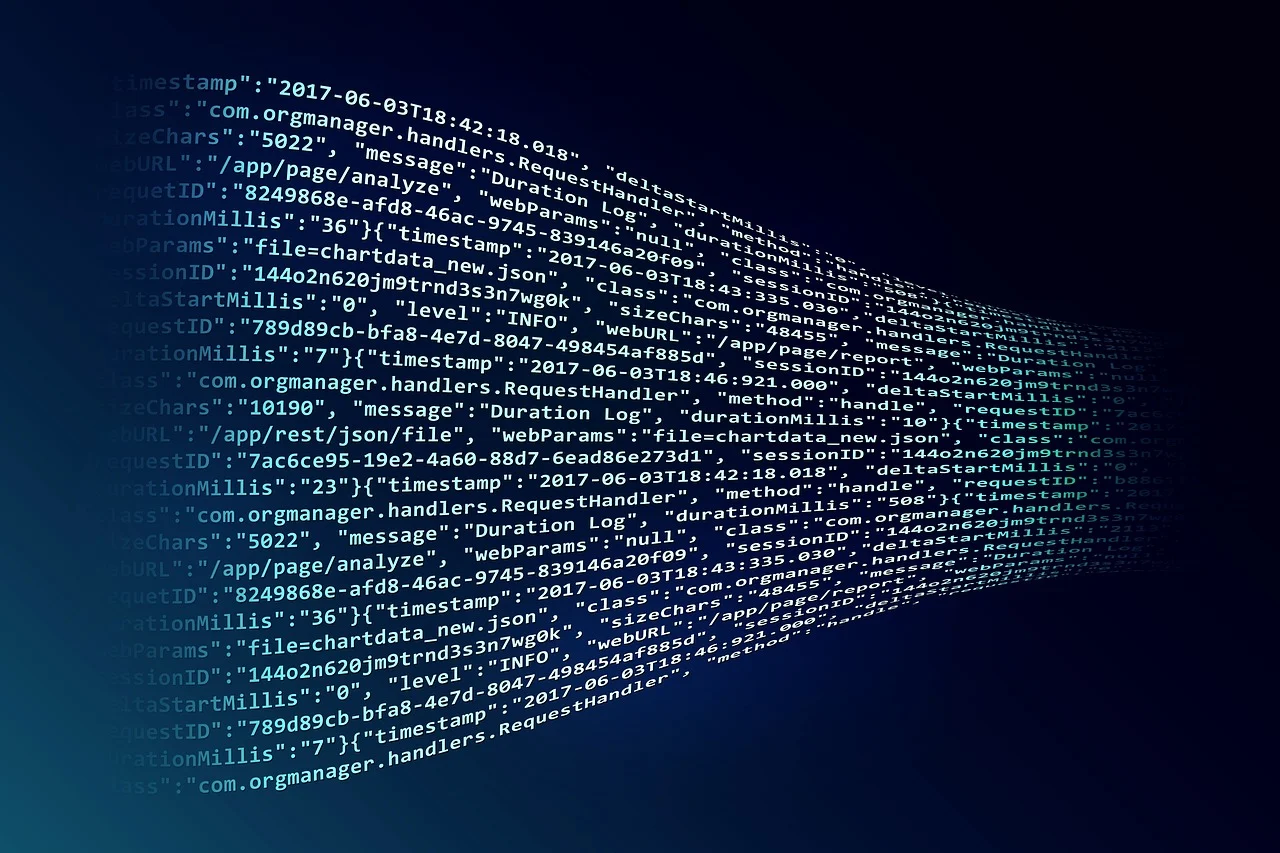
Log Parsing and Filtering
Log files often contain a vast amount of data, making it essential to parse and filter the information to focus on specific events or errors. Log parsing involves breaking down log entries into individual fields for easier analysis. Various tools and programming languages provide log parsing capabilities, allowing you to extract valuable information such as timestamps, error messages, IP addresses, and more.
Once the logs are parsed, filtering techniques can be employed to narrow down the data set based on specific criteria. Filtering can be performed using regular expressions, keywords, or specific log levels. By eliminating irrelevant data, you can focus your analysis on the critical events or errors that require attention.
Log parsing is the process of breaking down log entries into individual fields or elements for easier analysis. Log files are typically structured, with each log entry containing specific information such as timestamps, log levels, error codes, IP addresses, and more. By parsing logs, you extract these relevant fields, making it easier to analyze and interpret the log data.
Visualizing Log Data
Log analysis becomes more manageable and insightful when data is presented in a visual format. Data visualization tools enable you to create charts, graphs, and dashboards that provide a clear overview of log data patterns and trends. Visualization helps identify anomalies, spot recurring issues, and monitor system performance.
Popular tools like Kibana, Grafana, and Tableau allow you to create interactive visualizations that can be shared with teams, facilitating collaboration and enabling faster decision-making based on log insights.
Visual representations such as line charts, bar graphs, and pie charts can provide a clear overview of log data patterns and trends. Line charts are useful for tracking changes over time, while bar graphs can help compare different log events or error frequencies. Pie charts are effective for representing log distributions or percentages.
Correlation and Cross-Referencing
Logs from different sources or components of a system can be correlated and cross-referenced to gain a comprehensive understanding of complex events or incidents. Correlation involves analyzing logs from multiple systems or applications simultaneously to identify relationships and dependencies.
By cross-referencing logs, you can trace the flow of events across different components, enabling you to pinpoint the root cause of an issue. This approach is particularly useful in distributed systems, where logs from various microservices need to be analyzed collectively.
Correlation and cross-referencing log data involve analyzing logs from different sources or components to identify relationships and dependencies. This approach allows you to gain a comprehensive understanding of complex events or incidents.
Statistical Analysis
Statistical analysis techniques can be applied to log data to identify patterns, trends, and outliers. Statistical methods such as mean, median, standard deviation, and regression analysis can provide insights into system behavior, performance degradation, or anomalies.
By examining statistical measures, you can establish baselines for normal system behavior and detect deviations from these baselines. This approach helps in proactive monitoring, alerting you to potential issues before they escalate.
Statistical analysis plays a crucial role in log analysis as it helps identify patterns, trends, and anomalies within log data. By applying statistical methods, you can gain insights into system behavior, performance degradation, and potential issues.
Machine Learning for Log Analysis
Machine learning algorithms can be leveraged to automate log analysis tasks and enhance the detection of anomalies or patterns in log data. By training models on historical log data, machine learning algorithms can learn normal system behavior and identify abnormal events or outliers.
Techniques such as clustering, anomaly detection, and classification can be applied to log data to categorize logs, identify recurring issues, and detect security breaches. Machine learning-powered log analysis can significantly reduce manual effort and provide real-time insights into system health and performance.
Machine learning (ML) techniques have become increasingly popular for log analysis due to their ability to automate tasks, detect anomalies, and uncover patterns within log data. ML algorithms can learn from historical log data and make predictions or identify abnormal events in real-time
Real-time Monitoring and Alerting
Real-time monitoring and alerting systems ensure that critical events or errors are promptly addressed. By implementing tools or frameworks that continuously monitor logs, you can set up alerts for specific log patterns, error messages, or performance thresholds.
Real-time monitoring enables immediate response to issues, minimizing downtime and reducing the impact on users. It provides the ability to track system metrics in real-time and react proactively to potential issues.
Real-time monitoring and alerting are essential components of log analysis, enabling prompt response to critical events or errors. By continuously monitoring logs and setting up alerts, you can quickly address issues, minimize downtime, and ensure the smooth operation of your systems.
Log Aggregation and Centralized Logging
In environments with multiple systems and applications, log aggregation and centralized logging become crucial for efficient log analysis. Log aggregation involves collecting logs from various sources and consolidating them into a central repository. This approach simplifies the log analysis process by providing a single source of truth.
Centralized logging solutions like Elasticsearch, Splunk, or Graylog offer powerful features for collecting, indexing, and searching logs across distributed systems. With centralized logging, you can easily access and analyze logs from different components, enabling comprehensive analysis and troubleshooting.
Log aggregation and centralized logging are crucial practices in log analysis, especially in complex environments with multiple systems and applications. These techniques involve collecting logs from various sources and consolidating them into a central repository for efficient analysis and troubleshooting.
Historical Log Analysis
Analyzing historical log data can unveil long-term trends, recurring patterns, and historical issues. By reviewing historical logs, you can identify recurring errors, performance bottlenecks, or security breaches that may have gone unnoticed. Historical log analysis helps in identifying systemic issues and implementing long-term solutions to improve overall system stability.
Tools that provide log retention and archiving capabilities are valuable for storing and accessing historical log data. By maintaining a comprehensive log history, you can perform in-depth analysis, identify patterns, and make data-driven decisions for system improvements.
Historical log analysis involves analyzing log data from past time periods to gain insights into system behavior, identify recurring patterns, and address historical issues. It plays a crucial role in understanding long-term trends, detecting hidden problems, and implementing preventive measures
Collaborative Log Analysis
Log analysis is often a collaborative effort involving multiple teams, such as developers, operations, and security personnel. Collaborative log analysis allows different teams to share insights, observations, and expertise, leading to faster problem resolution and improved system performance.
Tools that facilitate collaboration, such as shared dashboards, annotations, and comment features, promote effective communication and knowledge sharing. Collaborative log analysis encourages cross-functional teams to work together, leveraging their collective expertise to analyze logs comprehensively.
Collaborative log analysis involves the collective effort of multiple teams or stakeholders to analyze and interpret log data. It promotes effective communication, knowledge sharing, and cross-functional collaboration, leading to faster problem resolution and improved system performance
Continuous Improvement and Iterative Analysis
Log analysis is an iterative process that requires continuous improvement. As you gain insights from log analysis, it is important to implement changes, monitor their impact, and iterate on your analysis techniques.
Regularly revisiting your log analysis approach, refining queries, adjusting alert thresholds, and incorporating new tools or technologies can enhance the effectiveness of your log analysis efforts. By embracing a continuous improvement mindset, you can optimize your log analysis process and stay ahead of evolving system challenges.
Continuous improvement and iterative analysis are essential practices in log analysis that ensure ongoing optimization of the log analysis process. By regularly revisiting and refining your log analysis approach, you can enhance the effectiveness of your analysis techniques and stay ahead of evolving system challenges
Conclusion: Logs contain a wealth of information that can drive improvements in system performance, security, and troubleshooting. By employing effective log analysis techniques, such as log parsing, visualization, correlation, statistical analysis, machine learning, and real-time monitoring, you can unlock valuable insights hidden within your log files. Understanding how to analyze logs using the file empowers you to make informed decisions, enhance system performance, and ensure a seamless user experience.
Analyzing logs using the file is a powerful approach to gain valuable insights into system behavior, troubleshoot issues, and ensure optimal performance. By employing techniques such as log parsing, visualization, correlation, statistical analysis, machine learning, real-time monitoring, log aggregation, historical analysis, collaborative analysis, and continuous improvement, you can harness the full potential of log files. Embrace these effective ways to analyze logs, and empower yourself to make data-driven decisions, enhance system reliability, and provide a seamless user experience.
We realised we really wanted to catch a glimpse of what went on behind the scenes of the companies we looked up to. And we thought other people would want to know too.
So we decided to organise an event to share these stories. Today, we run monthly Show & Tell events and an annual conference.